AI Governance Best Practices
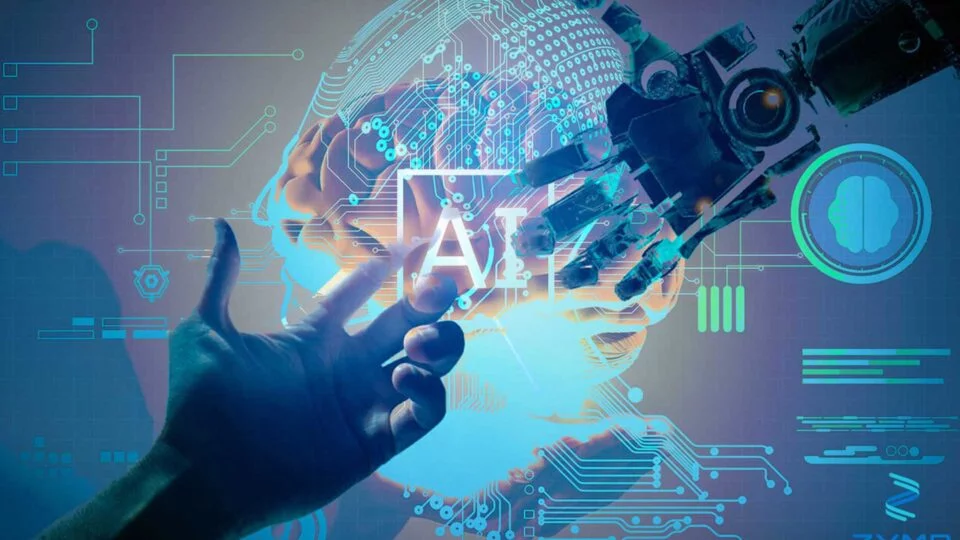
AI Governance Best Practices
1. Managing Risk:
AI systems can introduce various risks, including biased decision-making, security vulnerabilities, and legal liabilities. To effectively manage risk in AI governance, organizations should conduct thorough risk assessments during AI development and deployment. This involves identifying potential risks, such as algorithmic bias, and implementing strategies to mitigate them. Regular monitoring and auditing of AI systems are also crucial to detect and address emerging risks. Additionally, organizations should have clear protocols for handling incidents and breaches related to AI to minimize harm and legal consequences.
2. Maintaining Trust:
Trust is a fundamental aspect of AI governance. Users and stakeholders must have confidence that AI systems will behave ethically, reliably, and securely. To maintain trust, organizations should be transparent about their AI systems’ capabilities and limitations. They should also provide clear explanations for AI-driven decisions and ensure that their algorithms are fair and unbiased. Building trust also requires robust data protection and security measures to safeguard sensitive information and prevent misuse.
3. Building Internal Governance Structures:
Establishing internal governance structures is essential for effective AI governance. This involves defining roles and responsibilities within an organization, designating AI ethics committees or task forces, and ensuring that AI projects are supervised by qualified professionals. These structures help in making informed decisions, aligning AI initiatives with organizational values, and promoting accountability in AI development and deployment.
4. Engaging Stakeholders:
Engaging stakeholders, including customers, employees, regulators, and the public, is crucial in AI governance. Organizations should seek input and feedback from these groups to ensure that AI systems meet their expectations and needs. Transparency in AI decision-making processes, such as algorithmic transparency, can help build trust among stakeholders.
5. Evaluating AI’s Human Impact:
AI’s impact on society, particularly on human lives and well-being, should be carefully evaluated as part of AI governance. This involves considering the potential social, ethical, and economic consequences of AI systems. Organizations should assess how AI affects employment, privacy, and individual rights, and take measures to mitigate negative impacts.
6. Managing AI Models:
Effective AI governance includes robust management of AI models throughout their lifecycle. This entails ongoing monitoring, updating, and auditing of AI models to ensure they remain accurate, unbiased, and safe. Organizations should establish clear procedures for model version control, testing, and deployment, along with mechanisms for model retraining as new data becomes available.
7. Addressing Data Governance and Security:
Data is the foundation of AI, and data governance is a critical aspect of AI governance. Organizations should establish data governance policies that cover data quality, privacy, security, and compliance with relevant regulations (e.g., GDPR or CCPA). Secure storage and handling of data, as well as data access controls, are essential to protect sensitive information and ensure compliance with data protection laws.
In summary, AI governance is essential to ensure responsible and ethical AI development and deployment. These best practices help organizations manage risks, maintain trust, and align AI initiatives with societal values and expectations.