AI Bias and Ensuring Fairness in Machine Learning Models
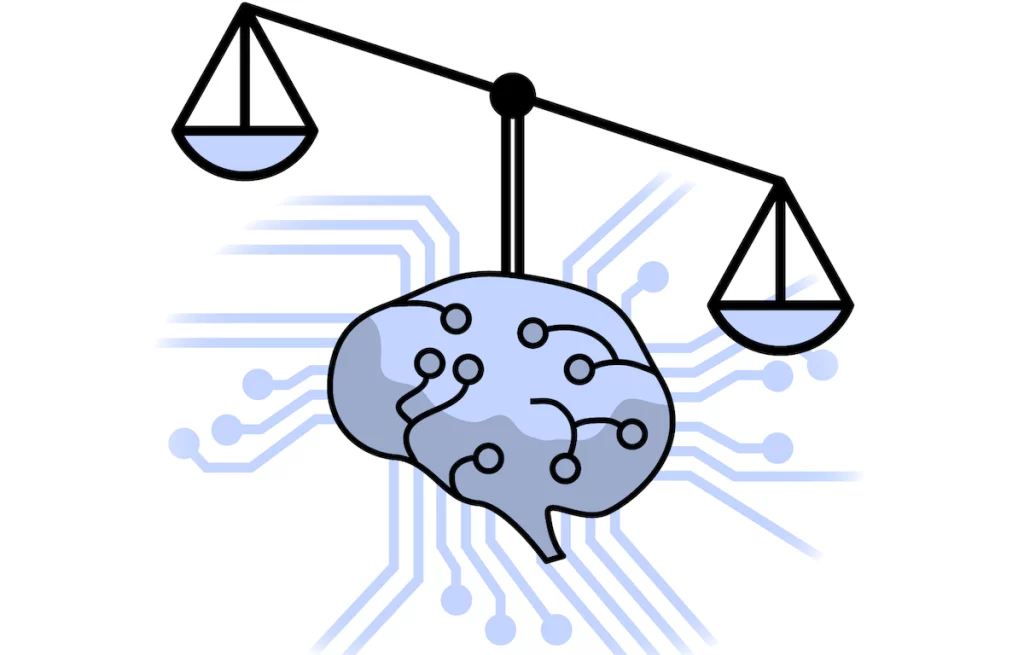
bias-and-fairness-in-ai
As artificial intelligence (AI) systems play an increasingly significant role in our daily lives, it becomes crucial to address AI bias and ensure fairness in machine learning models. Bias in AI refers to the presence of unfair, discriminatory, or skewed behaviors in the decisions and predictions made by AI algorithms. This bias can have profound real-world implications, such as reinforcing societal inequalities or causing harm to certain groups. To address these issues, we must understand where AI bias originates, how to measure it, assess AI fairness, and effectively mitigate bias.
What Does It Mean for an AI Model to Be “Biased”?
AI bias refers to the unjust and prejudiced treatment of different groups or individuals by an AI model. Bias can manifest in various ways, including inaccuracy, underrepresentation, or overrepresentation of specific groups, resulting in unequal opportunities or discriminatory outcomes.
Where Does AI Bias Come From?
AI bias often stems from the data used to train machine learning models. Biased training data, which reflects historical inequalities and stereotypes, can perpetuate those biases in the AI system’s predictions and decisions. Additionally, bias can be introduced during the model’s design, algorithm selection, or even through the biases of the human developers.
How Do I Measure AI Bias?
Measuring AI bias involves analyzing model outputs to identify disparities in performance across different groups. Common metrics include disparate impact, equal opportunity, and statistical parity. Additionally, qualitative assessments may involve examining the model’s predictions and the potential social and ethical implications they carry.
How Do I Measure AI Fairness?
AI fairness measurement evaluates the fairness of model outcomes and decisions. Techniques include demographic parity, predictive parity, and disparate mistreatment analysis. Evaluating fairness helps ensure that AI systems do not disproportionately harm or favor specific groups.
How Do I Mitigate AI Bias?
Mitigating AI bias is an ongoing process that begins with collecting and curating unbiased training data. Algorithmic techniques, such as re-weighting data or adjusting model parameters, can help reduce bias. Regular audits and monitoring of model performance are essential to detect and address bias in real-world applications continually. Furthermore, involving diverse perspectives in AI development and conducting ethical impact assessments can help promote fairness and minimize bias.
Conclusion:
Addressing AI bias and ensuring fairness in machine learning models are essential steps in building responsible and ethical AI systems. By understanding the origins of bias, measuring it, assessing fairness, and implementing mitigation strategies, we can work towards creating AI models that make equitable decisions and contribute positively to society.